
산업용 검사장비 비전 센서 기술 동향
1한국광기술원 지능형광학모듈연구센터Copyright © The Korean Society for Precision Engineering
This is an Open-Access article distributed under the terms of the Creative Commons Attribution Non-Commercial License (http://creativecommons.org/licenses/by-nc/3.0) which permits unrestricted non-commercial use, distribution, and reproduction in any medium, provided the original work is properly cited.
Abstract
The fourth industrial revolution is rapidly emerging as a new innovation trend for industrial automation. Accordingly, the demand for inspection equipment is highly increasing and vision sensor technologies are continuously evolving. Machine vision algorithms applied to deep learning are also being rapidly developed to maximize the performance of inspection equipment. In this review, we highlight the recent progress of vision sensor technology for the industrial inspection system. In particular, inspection principles and industrial applications of a vision sensor are classified according to the vision scanning methods. We also discuss machine vision-based inspection techniques containing rule- and deep learning-based image processing algorithms. We believe that this review provides novel approaches for various inspection fields of agriculture, medicine, and manufacturing industries.
Keywords:
Line scan vision sensor, 2D vision sensor, 3D vision sensor, Machine vision, Industrial inspection system키워드:
라인스캔 비전 센서, 2차원 비전 센서, 3차원 비전 센서, 머신 비전, 산업용 검사 시스템1. 서론
4차 산업혁명(Fourth Industrial Revolution, 4IR) 시대가 빠르게 도래됨에 따라 제조 및 물류 분야에서 자동화 및 무인화가 급증하고 있으며, 이에 따라 검사장비의 중요성은 지속적으로 증가하고 있다.1-3
검사장비의 정확도를 증대시키기 위하여 인공지능기술과 같은 지능형 알고리즘과 결합한 지능형 검사 시스템이 개발되고 있다. 이러한 지능형 검사 시스템을 통하여 생산성과 효율성을 높이기 위한 연구들이 진행되고 있으며, 급변하는 신제품들을 높은 품질로 빠르게 공급하기 위해 산업용 검사장비 기술의 중요성이 확장되고 있다.4,5
지능형 검사 시스템은 또한 비접촉 측정이 가능하며 제조 공정 또는 물류이동 시 즉각적인 피드백을 제공하여 다양한 작업 환경에서 적용 가능하다는 이점이 있다. 머신 비전 또는 비전 센서는 이미 스마트 팩토리, 스마트팜 등을 비롯한 다양한 영역에 중요한 부분으로 적용되고 있으며, 이 기술들은 점진적으로 지능화되어 자가 학습을 통한 새로운 환경에 대응이 가능하다.6,7
지능형 검사 시스템은 질량, 속도, 온도, 전기장 세기 등 기본적인 물리량을 측정하는 비전 센서 및 획득된 영상 이미지 데이터베이스를 관리하고 처리하는 영역들을 포함한다. 비전 센서기술은 영상 이미지를 제공하는 카메라를 넘어 크기, 색상, 위치 및 패턴 비교에 필요한 파라미터들을 수집하는 다기능 센싱 분야로 발전되고 있으며, 조명에서 발생된 빛이 검사 대상물에 도달 후 변화되는 정보 또한 수집된다.8
구체적으로 이미지 획득을 위한 비전 센서는 이미지 센서, 영상처리 장치 및 광학계로 구분이 가능하며 응용분야에 따라 다양한 차원의 이미징을 할 수 있는 광학계로 구성할 수 있다. 이미지 센서에 결상된 빛은 전기적인 신호로 변경되어 이미지 프로세서를 통해 디지털 영상으로 출력되고, 출력된 이미지는 머신 비전과 같은 영상처리 기법을 통하여 대상물을 분석하는데 사용된다.9,10
머신 비전 또는 컴퓨터 비전 알고리즘은 스마트 팩토리, 자율주행 등과 같은 자동화 분야에서 매우 중요한 역할을 수행한다.11-13
머신 비전은 비전 센서를 통하여 획득한 이미지에서 목표 객체를 인식하고 분석함으로 자동 검사, 로봇 가이드 등을 수행하는 분야이다.14,15
머신 비전 방법은 획득한 영상 정보를 직관적으로 분석할 수 있어 높은 처리량의 검사가 가능하며, 인적 오류를 감소시켜 높은 정밀도를 가지는 검사장비 구현에 도움을 준다. 머신 비전 기술은 객체 인식 알고리즘을 통하여 제품의 불량 검사, 치수 측정, 바코드 인식 등 다양한 자동화 기술에 적용되고 있으며, 반도체 소자, 자동차부품 및 식품 등 적용 분야가 확대되고 있다.16-18
본 논문에서는 자동화 시스템 구현에 효율적인 전략을 제시하기 위한 산업용 검사장비 비전 센서 기술 동향 분석을 제공한다. 전체적인 논문의 구성은 비전 센서의 동작 방식에 따라서 1차원, 2차원 및 3차원 스캔 방식 구분되어 있으며, 마지막 절에서는 머신 비전 응용을 소개한다(Fig. 1(a)). 각 절에서는 비전 센서의 다양한 스캔 방식들을 소개하고, 응용분야에 적용된 사례연구를 제시한다. 규칙(Rule) 기반 검사 기술과 딥러닝 기반 검사 기술로 구분한 머신 비전 응용 연구 방법들 또한 소개 한다(Fig. 1(b)). 그리고 결론에서는 산업용 비전 센서의 수요 분야와 향후 발전 전망에 대하여 언급한다.
2. 라인스캔 비전 센서
2.1 TDI (Time Delayed Integration) 라인스캔 비전 센서
라인스캔 비전 센서는 이미지 센서가 라인으로 구성되어 있는 것이 특징이며, 인쇄회로기판(Printed Circuit Board, PCB), 디스플레이 패널 및 직물과 같이 길이가 길며 연속적인 검사에 적용된다(Fig. 2(a)). 라인스캔 비전 센서를 이용한 검사는 물체를 일정한 속도로 움직이면서 측정하여 이미지 데이터를 획득하며, 생산성 증대를 위해서는 빠른 속도로 선명한 고해상도의 이미지를 얻는 것이 필요하다. 일반적인 라인스캔의 이미지 센서는 아래 Fig. 2(a)에서 나타내는 것과 같이, 한쪽 방향으로 정렬된 픽셀이 한 줄로 구성되고 기본 사양은 Pixel 수/Row로 표현된다. 라인스캔의 해상도는 획득된 광학 정보의 양에 의해 결정되며, 샘플에 조사되는 광원의 세기와 비례 관계, 스캔속도와는 반비례 관계를 가지고 있다. 생산성을 고려하여 광원의 세기 및 스캔속도와 관계없이 광학정보의 양을 늘리기 위해서 Fig. 2(b)에서 보는 것과 같이 여러 개의 라인 센서를 나란히 배열하여 사용하고 있다. TDI 라인스캔 비전 센서의 각 이미지 센서에서는 시간 간격에 따라서 광학 정보를 순차적으로 획득하고, 이미지 프로세싱을 통해서 이미지를 재구성하게 된다. 일반적인 라인스캔 비전 센서에 비해 획득되는 광학 정보의 양이 많기 때문에 노이즈의 양이 감소하며 고해상도 이미지를 획득할 수 있다(Fig. (2)).19
2.2 컬러(Color) 라인스캔 비전 센서
라인스캔 비전 센서는 한 줄의 이미지 센서만을 가지고 있기 때문에 대상물의 색상에 정보를 획득할 수 없다. 대상물의 색상을 표현하기 위해서 RGB (R: Red, G: Green, B: Blue) 컬러 센서가 필요하며 이를 위해 다양한 형태의 이미지 센서가 제안되었다.20
프리즘 라인스캔 비전 센서는 광학 정보를 수집하는 광학계와 수집된 광학 정보를 파장별로 분리하는 Beam Splitter Prism 및 이미지 센서로 구성되어 있다(Fig. 3(a)).21
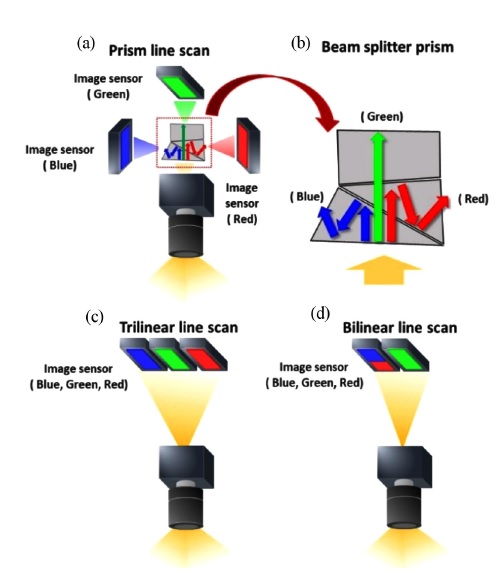
Schematic illustrations of (a) Prism line scan vision sensor composed of color image sensors (Blue, green, red), and (b) Beam splitter prism. The color line scan vison sensor without prism categorized with (c) trilinear line scan, and (d) bilinear line scan respect to the pixel size
Beam Splitter Prism은 아래 Fig. 3(b)에서 나타내는 것과 같이 첫 번째 프리즘에서는 파란색에 해당되는 파장만을 반사하고, 두 번째 프리즘에서는 빨간색에 해당되는 파장만을 반사하며 최종적으로는 녹색에 해당되는 파장만을 통과하도록 하는 프리즘 조합으로 구성되어 있다. 특정 파장을 반사 또는 투과하기 위해 입사광에 대한 프리즘의 각도 및 코팅 규격이 결정된다. 프리즘에서 분리된 파장별 광학 정보는 각각의 파장 대역에 해당되는 이미지 센서(R, G, B)에 수집된 후 이미지 프로세싱을 통하여 최종 영상을 획득하는데 사용된다. 이 경우에는 프리즘의 구조에 따라 광경로 및 초점거리가 달라지기 때문에 광학계 정렬에 대한 어려움이 있으며, 센서 모듈의 크기가 커지는 단점을 가지고 있다. Trilinear 라인스캔 비전 센서의 경우 Red, Green, Blue 컬러 센서 3줄을 차례로 구성하여 이미지를 구성하는 방식으로 센서 위치의 차이를 보정하는 기술이 필요하며, 대상물을 이동시켜 측정한 뒤 융합하여 최종 이미지를 획득한다 (Fig. 3(c)).22
Trilinear 라인스캔 센서는 프리즘 라인스캔 비전 센서에 비해 작은 크기와 단일 광학계를 사용한 비용 절감의 장점을 가지고 있다. Bilinear 라인스캔 비전 센서는 Trilinear 라인스캔 비전 센서와 달리 2줄의 이미지 센서를 가지고 있기 때문에 위치에 따른 보정이 상대적으로 수월하나 각 색상별 픽셀의 크기에 따른 획득효율로 인한 이미지 보상 작업이 필요하다(Fig. 3(d)).23
3. 2차원 스캔 비전 센서
2차원 스캔 비전 센서는 전통적으로 산업용 검사장비에 가장 많이 활용되는 방법으로 비전 센서가 장착된 스테이지를 측정 대상물로 이동하여 이미지를 얻는 방법과 고정된 비전 센서 아래로 대상물을 이동시켜 특정 영역을 측정하는 두 가지 방법으로 구분된다. 검사 영역 및 이미지의 해상도는 적용된 광학계의 시야각(Field of View, FOV)과 센서의 픽셀 규격에 의존한다. 라인스캔 비전 센서에 비해 측정 속도가 상대적으로 느리지만 높은 선명도를 갖는 것이 일반적이며, 이러한 단점을 해결하기 위해서 고속으로 대면적으로 이미지를 측정한 뒤, 불순물 등의 정밀 스캔이 필요한 영역은 줌 기술을 이용하여 확대하여 측정하는 방법을 적용하고 있다(Fig. 4).24
4. 3차원 스캔 비전 센서
4.1 스테레오 비전 기술
스테레오 비전 기술이란 2개 이상의 카메라들을 이용하여 동일한 물체를 다른 방향에서 촬영 후 물체의 3차원 정보를 획득하는 방법이다(Fig. 5(a)).25
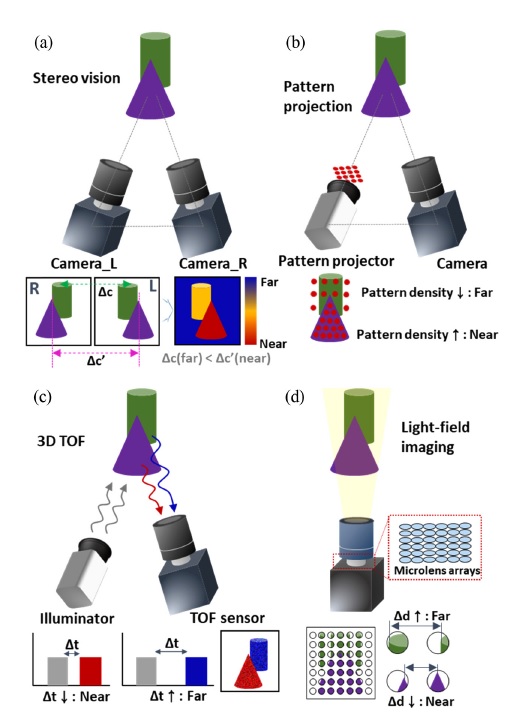
Schematic illustrations of 3D scan vision sensor. (a) 3D imaging through stereo vision. (b) Acquisition of 3D information by using pattern projection, (c) 3D TOF sensor for 3D depth estimation, and (d) 3D surface imaging based on alight field camera
3차원 깊이 정보를 획득하기 위해서는 여러 카메라들로 획득된 이미지들을 정렬시킨 후 대응점들을 정합(Matching)시켜 대상물의 거리감을 예측한다.26
이러한 대응점들의 차이를 디스패리티(Disparity)라고 하며 디스패리티 값의 차이로 거리를 계산할 수 있다. 예를 들어 원거리에 있는 물체는 두 이미지들 간의 동일한 위치에 결상되어 디스패리티가 작으며, 근거리에 있는 물체는 두 이미지들 간의 결상된 위치 차이가 커 근거리에 있음을 분석할 수 있다. 이러한 방법은 상대적으로 특징점들이 적은 평평한 3차원 면들을 가진 물체의 경우 픽셀 차이 계산이 어려워 정확도가 떨어진다는 한계가 있다. 하지만 카메라를 이용하여 상대적으로 높은 해상도의 이미지를 획득하기 때문에 제품의 형태 불량 및 표면 결함 검사에 주로 이용된다. 이러한 방법을 이용하여 산업용 로봇의 반복적인 작업에 대한 작동 신뢰성을 향상시키기 위한 주기적인 교정(Calibration)작업을 수행한 연구가 소개되었다.27
이 방법은 스테레오 비전 기법을 통하여 시각적 매칭을 통하여 로봇의 좌표계를 보정하여 반복적인 작동에 대한 신뢰성을 높이는 기능을 수행할 수 있다.
4.2 패턴 프로젝션 기술
패턴 프로젝션 기술은 특정 패턴을 가진 조명을 물체에 조사한 후 패턴이 변형된 정보를 기하학적으로 분석하여 깊이 정보를 획득한다(Fig. 5(b)).28,29
패턴 프로젝션 기술에 이용되는 조명은 점 어레이(Dot Array), 스페클 패턴(Speckle Pattern), 선 어레이, 사인파 프린지(Sinusoidal Fringe), 모아레 패턴(Moire Pattern) 등이 있으며, 주로 패턴들은 공간 광 변조기(Spatial Light Modulator, SLM)를 통하여 생성된다.30-33
이 방법은 빛 반사의 한계로 인하여 깊이가 깊은 물체는 측정하기 어렵다는 단점이 있지만, 수 μm 정도의 깊이 해상력을 구현할 수 있어 인쇄 회로 기판 및 미세 구조물 검사 등에 주로 이용된다.34
또한, 물체의 질감 및 반사도에 따라서 패턴의 모양 또는 세기가 달라지기 때문에, 물체의 표면 특성을 검출하는 응용 분야에서도 패턴 프로젝션 기술이 사용된다.35
4.3 3차원 비행시간(Time of Flight, TOF) 검사 기술
3차원 TOF 방식은 물체에 반사된 빛의 시간 차이를 통하여 3차원 정보를 획득한다(Fig. 5(c)).36
조명부에서 펄스 광원을 물체에 전달하고 TOF 센서에서 반사된 신호의 세기 및 시간 변화를 이용하여 표면 형상을 계산한다. TOF 센서는 시간분해능이 좋아 매우 빠른 관측이 필요하거나 물체가 넓은 영역에 위치 할 경우 주로 사용된다. 이러한 특징으로 인하여 TOF 센서는 주로 자율 주행을 위한 센서로 많이 이용될 뿐 아니라 화물 운반 및 포장 분야에도 사용된다. 화물의 포장 및 운반을 위해서는 물건, 포장 박스, 운송체 등의 위치 및 높이를 정확하게 계산하는 것이 필요하다.37
TOF 센서는 다양한 물체들의 깊이 정보를 파악하여 정확한 위치로 이송되는 자동 운송 시스템에 이용되고 있다.38,39
4.4 라이트필드(Light-Field) 이미징 기술
라이트필드 이미징 기술은 하나의 영상 시스템을 통하여 3차원 이미지를 획득하는 방식이다(Fig. 5(d)).40-42
라이트필드 카메라에는 주로 미세 렌즈 배열(Microlens Arrays)이 포함되어 있어 한번의 촬영으로 각 렌즈의 채널마다 서로 다른 공간적 정보를 획득한다.43
각 채널에서 획득된 정보들을 재구성함으로서 3차원 깊이 이미징, 리포커싱(Refocusing) 등의 기능들을 구현 가능하다.44
이러한 라이트필드 카메라는 전체적인 시스템을 소형화가 가능하고, 피사계 심도가 매우 넓다는 장점을 가지고 있다. 상업용 라이트필드 카메라를 최초로 개발한 Raytrix GmbH는 커넥터핀, 본딩 와이어 등의 회로 부품뿐 아니라 표면 홈 또는 스크래치의 3차원 깊이 이미지를 획득하였다.45
또한, 물체의 상대적인 위치를 파악하는 시각적 오도메트리(Visual Odometry) 응용에도 라이트필드 카메라가 이용되었다.46
5. 머신 비전 응용
5.1 규칙(Rule) 기반 검사 기술
규칙 기반의 검사 기술이란 전통적으로 제조 검사장비에서 사용되던 방식으로 변수를 직접 사용자가 입력하여 이미지를 분석하는 방식이다.52,53
이러한 방식을 바코드, 레이블, 2D 매트릭스 코드 등 정해진 식별 코드를 빠르게 인식하는데 유리하다. 또한 반복적인 패키징 공정 시에 정해진 부품의 위치와 방향을 찾고 지정된 오차 내의 거리로 이동시키는 작업을 수행할 때 이용된다. 하지만, 정량화가 가능한 데이터를 인식하는 단순 검사 이외에 정성적 판단이 요구되는 품질검사 분야에서는 정확도가 매우 낮고, 설정된 규칙에 따라 오차가 높다는 단점이 있다. 특히, 규칙 기반 검사를 통하여 비정형의 이미지를 통하여 품질을 검사하는 방법은 규칙을 설정하기가 매우 어렵고 제품이 변경될 때마다 새로운 검사 판정 기준을 설정해야 하는 문제가 있어 전체적인 유지비용이 증가된다는 한계점을 가지고 있다.
5.2 딥러닝 기반 검사 기술
기존의 규칙 기반의 검사 기술의 한계를 극복하고자 사례를 기반으로 학습한 데이터를 통하여 검사를 수행하는 기술이 도입되었다.54
딥러닝 기반 검사 기술은 미리 학습시킨 이미지 예제들과 인공신경망을 기초하여 제품의 정보검색 및 불량 여부 등을 판단하는 역할을 수행할 수 있다(Fig. 6).55
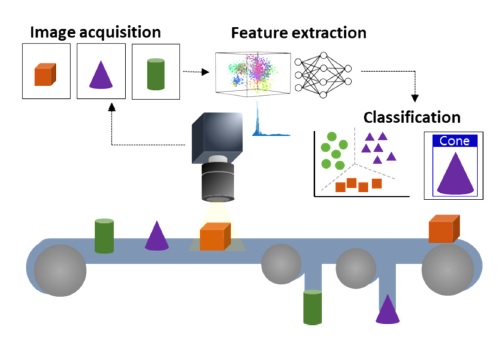
A schematic illustration of deep-learning based inspection system. After capturing images through vision sensor, feature points are extracted by image processing algorithms. The inspection system finally estimates the object through the classification of trained data
예를 들어 이물, 스크래치, 얼룩과 같은 불량 검출이나 부품 유무, 포장 상태, 음료의 유량 등의 검사에 활용 가능하다.56-58
이러한 딥러닝 기술은 제품의 크기 또는 위치가 변경되어도 재프로그래밍 없이 레퍼런스 이미지들만 변경하여 적용이 가능하다는 특징을 가지고 있다. 또한, 새로운 검사 기준을 수립할 때에도 이미 훈련된 신경망을 이용하면 빠른 대응이 가능하고 높은 신뢰성을 갖는 검사가 가능하여 유지 비용을 절감시킬 수 있다는 장점이 있다.
6. 결론
본 논문에서는 산업용 검사장비 비전 센서 기술을 스캐닝 방법과 머신 비전 검사 기술에 따라 구분하여 소개하여 보았다. 검사용 비전 센서는 획득되는 이미지들이 포함하는 정보들의 구성에 따라서 1D, 2D 및 3D 비전 센서로 구분하였으며, 응용분야에 따라 다양한 구성의 비전 센서가 적용되고 있다. 검사의 정확도 및 처리량 증대를 위하여 비전 센서의 해상도가 지속적으로 향상되고 있으며, 빠르게 대상물을 판단하기 위한 고속 측정 기술도 발전되고 있다. 특히, 반도체 패턴과 같이 크기가 지속적으로 미세화되는 경우 더 높은 해상도의 광학 검사장비가 필요하며, 최근 수요가 증가하는 2차 전지 소자의 불량 검사에도 광학 검사는 필수적인 요소로 손꼽히고 있다. 또한, 최근 딥러닝 기술의 발전으로 머신 비전을 적용한 고지능화된 검사 방법이 다양한 분야로 확장되고 있다. 딥러닝 기술은 재프로그래밍 없이 사전 훈련된 데이터를 이용하여 검사를 할 수 있어 대응이 빠르지만, 초기 학습을 위한 많은 수의 정보를 필요로 하기 때문에 규칙 기반 검사 기술과의 비교를 통하여 영상처리 기법의 선정이 필요하다. 검사장비용 비전 센서 기술은 제조장비와 결합되어 실시간 모니터링 및 자동 피드백을 통하여 생산성 향상에 기여할 수 있을 것으로 기대하며, 여러 검사 기능들이 결합된 통합 모듈로 발전될 것으로 예상된다. 이러한 소프트웨어적인 발전 이외에도 다양한 광학적 정보를 획득 가능한 다기능 비전 센서도 지속적으로 발전될 것으로 기대된다. 특히, 다양한 파장을 획득할 수 있는 초분광 이미징은 식품의 신선도, 이물 검출 및 제품의 생화학적인 정보 등의 정보들을 분석 가능하기 때문에 식품 및 바이오 산업 분야에도 사용될 수 있을 것으로 예상된다.
Acknowledgments
This work was supported by the Technology Innovation program (Development of 40-80 mm Diameter Aspheric Lens and Module with Glass Molding Process, No. 20011304) funded by the Ministry of Trade, Industry & Energy (MOTIE, Korea) and a grant of the National Research Foundation of Korea (NRF) (No. 2021R1F1A1048603).
REFERENCES
-
Kalsoom, T., Ramzan, N., Ahmed, S., and Ur-Rehman, M., “Advances in Sensor Technologies in the Era of Smart Factory and Industry 4.0,” Sensors, Vol. 20, No. 23, Paper No. 6783, 2020.
[https://doi.org/10.3390/s20236783]
-
Semeniuta, O., Dransfeld, S., Martinsen, K., and Falkman, P., “Towards Increased Intelligence and Automatic Improvement in Industrial Vision Systems,” Procedia CIRP, Vol. 67, No. pp. 256-261, 2018.
[https://doi.org/10.1016/j.procir.2017.12.209]
-
Coffey, V. C., “Machine Vision: The Eyes of Industry 4.0,” Optics and Photonics News, Vol. 29, No. 7, pp. 42-49, 2018.
[https://doi.org/10.1364/OPN.29.7.000042]
-
Kim, D. H., Kim, T. J., Wang, X., Kim, M., Quan, Y. J., et al., “Smart Machining Process Using Machine Learning: A Review and Perspective on Machining Industry,” International Journal of Precision Engineering and Manufacturing-Green Technology, Vol. 5, No. 4, pp. 555-568, 2018.
[https://doi.org/10.1007/s40684-018-0057-y]
-
Lee, J. Y., Yoon, J. S., and Kim, B.-H., “A Big Data Analytics Platform for Smart Factories in Small and Medium-Sized Manufacturing Enterprises: An Empirical Case Study of a Die Casting Factory,” International Journal of Precision Engineering and Manufacturing, Vol. 18, No. 10, pp. 1353-1361, 2017.
[https://doi.org/10.1007/s12541-017-0161-x]
-
Wan, J., Yang, J., Wang, Z., and Hua, Q., “Artificial Intelligence for Cloud-Assisted Smart Factory,” IEEE Access, Vol. 6, No. pp. 55419-55430, 2018.
[https://doi.org/10.1109/ACCESS.2018.2871724]
-
Mavridou, E., Vrochidou, E., Papakostas, G. A., Pachidis, T., and Kaburlasos, V. G., “Machine Vision Systems in Precision Agriculture for Crop Farming,” Journal of Imaging, Vol. 5, No. 12, Paper No. 89, 2019.
[https://doi.org/10.3390/jimaging5120089]
-
Bloss, R., “Latest in Vision Sensor Technology As Well As Innovations in Sensing, Pressure, Force, Medical, Particle Size and Many Other Applications,” Sensor Review, Vol. 37, No. 1, pp. 7-11, 2017.
[https://doi.org/10.1108/SR-09-2016-0186]
-
Yang, S.-P., Seo, Y.-H., Kim, J.-B., Kim, H., and Jeong, K.-H., “Optical MEMS Devices for Compact 3D Surface Imaging Cameras,” Micro and Nano Systems Letters, Vol. 7, No. 1, pp. 1-9, 2019.
[https://doi.org/10.1186/s40486-019-0087-4]
-
González, A. B. and Pozo, J., “The Industrial Camera Modules Market: Market Review and Forecast until 2022,” PhotonicsViews, Vol. 16, No. 2, pp. 24-26, 2019.
[https://doi.org/10.1002/phvs.201970207]
-
Zancul, E., Martins, H. O., Lopes, F. P., and da Silva Neto, F. A., “Machine Vision Applications in a Learning Factory,” Procedia Manufacturing, Vol. 45, pp. 516-521, 2020.
[https://doi.org/10.1016/j.promfg.2020.04.069]
-
Schmidt, J., Marques, M. R., Botti, S., and Marques, M. A., “Recent Advances and Applications of Machine Learning in Solid-State Materials Science,” npj Computational Materials, Vol. 5, No. 1, pp. 1-36, 2019.
[https://doi.org/10.1038/s41524-019-0221-0]
-
Fujiyoshi, H., Hirakawa, T., and Yamashita, T., “Deep Learning-Based Image Recognition for Autonomous Driving,” IATSS Research, Vol. 43, No. 4, pp. 244-252, 2019.
[https://doi.org/10.1016/j.iatssr.2019.11.008]
-
Chen, A. I., Balter, M. L., Maguire, T. J., and Yarmush, M. L., “Deep Learning Robotic Guidance for Autonomous Vascular Access,” Nature Machine Intelligence, Vol. 2, No. 2, pp. 104-115, 2020.
[https://doi.org/10.1038/s42256-020-0148-7]
-
Li, L., Ota, K., and Dong, M., “Deep Learning for Smart Industry: Efficient Manufacture Inspection System with Fog Computing,” IEEE Transactions on Industrial Informatics, Vol. 14, No. 10, pp. 4665-4673, 2018.
[https://doi.org/10.1109/TII.2018.2842821]
-
Yuan-Fu, Y., “A Deep Learning Model for Identification of Defect Patterns in Semiconductor Wafer Map,” Proc. of the 30th Annual SEMI Advanced Semiconductor Manufacturing Conference, pp. 1-6, 2019.
[https://doi.org/10.1109/ASMC.2019.8791815]
-
Jiang, J., Cao, P., Lu, Z., Lou, W., and Yang, Y., “Surface Defect Detection for Mobile Phone Back Glass based on Symmetric Convolutional Neural Network Deep Learning,” Applied Sciences, Vol. 10, No. 10, Paper No. 3621, 2020.
[https://doi.org/10.3390/app10103621]
-
Ma, L., Xie, W., and Zhang, Y., “Blister Defect Detection based on Convolutional Neural Network for Polymer Lithium-Ion Battery,” Applied Sciences, Vol. 9, No. 6, Paper No. 1085, 2019.
[https://doi.org/10.3390/app9061085]
-
Hochman, G., Yitzhaky, Y., Kopeika, N. S., Lauber, Y., Citroen, M., et al., “Restoration of Images Captured by a Staggered Time Delay and Integration Camera in the Presence of Mechanical Vibrations,” Applied Optics, Vol. 43, No. 22, pp. 4345-4354, 2004.
[https://doi.org/10.1364/AO.43.004345]
- Brückner, P., Schlegel, A., Lemanzyk, T., and Weber, G., “Novel Approach on Optical Analysis and Multichannel Sorting of Grain Samples,” Proc. of the XXI IMEKO World Congress, Measurement in Research and Industry, pp. 1-4, 2015.
-
Lemstrom, G. F., “True RGB Line-Scan Camera for Color Machine Vision Applications,” Intelligent Robots and Computer Vision XIII: Algorithms and Computer Vision, Vol. 2353, pp. 494-502, 1994.
[https://doi.org/10.1117/12.188921]
-
Schrey, O., Brockherde, W., Nitta, C., Bechen, B., Bodenstorfer, E., et al., “Tri-Linear Color Multi-Linescan Sensor with 200 kHz Line Rate,” Solid-State Electronics, Vol. 125, pp. 220-226, 2016.
[https://doi.org/10.1016/j.sse.2016.05.012]
-
Bodenstorfer, E., Hasani, Y., Fürtler, J., Brodersen, J., and Mayer, K. J., “High-Speed Line-Scan Camera with Multi-Line CMOS Color Sensor,” Proc. of the IEEE Computer Society Conference on Computer Vision and Pattern Recognition Workshops, pp. 9-14, 2012.
[https://doi.org/10.1109/CVPRW.2012.6238888]
-
Roh, Y. J., Lee, D.-Y., Kim, M.-Y., and Cho, H., “Visual Inspection System with Flexible Illumination and Autofocusing,” Proc. of the Optomechatronic Systems III on International Society for Optics and Photonics, pp. 463-475, 2002.
[https://doi.org/10.1117/12.467381]
-
Chen, F., Chen, X., Xie, X., Feng, X., and Yang, L., “Full-Field 3D Measurement Using Multi-Camera Digital Image Correlation System,” Optics and Lasers in Engineering, Vol. 51, No. 9, pp. 1044-1052, 2013.
[https://doi.org/10.1016/j.optlaseng.2013.03.001]
-
Hosni, A., Rhemann, C., Bleyer, M., Rother, C., and Gelautz, M., “Fast Cost-Volume Filtering for Visual Correspondence and Beyond,” IEEE Transactions on Pattern Analysis and Machine Intelligence, Vol. 35, No. 2, pp. 504-511, 2012.
[https://doi.org/10.1109/TPAMI.2012.156]
-
Švaco, M., Šekoranja, B., Šuligoj, F., and Jerbić, B., “Calibration of an Industrial Robot Using a Stereo Vision System,” Procedia Engineering, Vol. 69, pp. 459-463, 2014.
[https://doi.org/10.1016/j.proeng.2014.03.012]
-
Zhang, S., “Recent Progresses on Real-Time 3D Shape Measurement Using Digital Fringe Projection Techniques,” Optics and Lasers in Engineering, Vol. 48, No. 2, pp. 149-158, 2010.
[https://doi.org/10.1016/j.optlaseng.2009.03.008]
-
Clancy, N. T., Stoyanov, D., Maier-Hein, L., Groch, A., Yang, G.-Z., et al., “Spectrally Encoded Fiber-Based Structured Lighting Probe for Intraoperative 3D Imaging,” Biomedical Optics Express, Vol. 2, No. 11, pp. 3119-3128, 2011.
[https://doi.org/10.1364/BOE.2.003119]
-
Salvi, J., Pages, J., and Batlle, J., “Pattern Codification Strategies in Structured Light Systems,” Pattern Recognition, Vol. 37, No. 4, pp. 827-849, 2004.
[https://doi.org/10.1016/j.patcog.2003.10.002]
-
Guo, H., Zhou, H., and Banerjee, P. P., “Single-Shot Digital Phase-Shifting Moiré Patterns for 3D Topography,” Applied Optics, Vol. 60, No. 4, pp. 84-92, 2021.
[https://doi.org/10.1364/AO.404424]
-
Li, E., Peng, X., Xi, J., Chicharo, J. F., Yao, J., et al., “Multi-Frequency and Multiple Phase-Shift Sinusoidal Fringe Projection for 3D Profilometry,” Optics Express, Vol. 13, No. 5, pp. 1561-1569, 2005.
[https://doi.org/10.1364/OPEX.13.001561]
-
Fu, K., Xie, Y., Jing, H., and Zhu, J., “Fast Spatial-Temporal Stereo Matching for 3D Face Reconstruction under Speckle Pattern Projection,” Image and Vision Computing, Vol. 85, No. pp. 36-45, 2019.
[https://doi.org/10.1016/j.imavis.2019.02.007]
-
Li, B., An, Y., Cappelleri, D., Xu, J., and Zhang, S., “High-Accuracy, High-Speed 3D Structured Light Imaging Techniques and Potential Applications to Intelligent Robotics,” International Journal of Intelligent Robotics and Applications, Vol. 1, No. 1, pp. 86-103, 2017.
[https://doi.org/10.1007/s41315-016-0001-7]
-
Xu, J., Xi, N., Zhang, C., Zhao, J., Gao, B., et al., “Rapid 3D Surface Profile Measurement of Industrial Parts Using Two-Level Structured Light Patterns,” Optics and Lasers in Engineering, Vol. 49, No. 7, pp. 907-914, 2011.
[https://doi.org/10.1016/j.optlaseng.2011.02.010]
-
He, Y., Liang, B., Zou, Y., He, J., and Yang, J., “Depth Errors Analysis and Correction for Time-of-Flight (ToF) Cameras,” Sensors, Vol. 17, No. 1, Paper No. 92, 2017.
[https://doi.org/10.3390/s17010092]
-
Monica, R., Aleotti, J., and Rizzini, D. L., “Detection of Parcel Boxes for Pallet Unloading Using a 3D Time-of-Flight Industrial Sensor,” Proc. of the 4th IEEE International Conference on Robotic Computing, pp. 314-318, 2020.
[https://doi.org/10.1109/IRC.2020.00057]
- Hussmann, S., Gonschior, M., Buttgen, B., Peter, C., Schwope, S., et al., “A Review on Commercial Solid State 3D Cameras for Machine Vision Applications,” Recent Advances in Topography Research, Vol. 1, pp. 303-351, 2013.
-
He, Y. and Chen, S., “Recent Advances in 3D Data Acquisition and Processing by Time-of-Flight Camera,” IEEE Access, Vol. 7, pp. 12495-12510, 2019.
[https://doi.org/10.1109/ACCESS.2019.2891693]
-
Bae, S. I., Kim, K., Jang, K. W., Kim, H. K., and Jeong, K. H., “High Contrast Ultrathin Light-Field Camera Using Inverted Microlens Arrays with Metal-Insulator-Metal Optical Absorber,” Advanced Optical Materials, Vol. 9, No. 6, Paper No. 2001657, 2021.
[https://doi.org/10.1002/adom.202001657]
- Ng, R., Levoy, M., Brédif, M., Duval, G., Horowitz, M., et al., “Light Field Photography with a Hand-Held Plenoptic Camera,” Stanford University Computer Science Tech Report, pp. 1-11, 2005.
-
Martínez-Corral, M. and Javidi, B., “Fundamentals of 3D Imaging and Displays: A Tutorial on Integral Imaging, Light-Field, and Plenoptic Systems,” Advances in Optics and Photonics, Vol. 10, No. 3, pp. 512-566, 2018.
[https://doi.org/10.1364/AOP.10.000512]
-
Cai, Z., Liu, X., Pedrini, G., Osten, W., and Peng, X., “Unfocused Plenoptic Metric Modeling and Calibration,” Optics Express, Vol. 27, No. 15, pp. 20177-20198, 2019.
[https://doi.org/10.1364/OE.27.020177]
-
Štolc, S., Soukup, D., Holländer, B., and Huber-Mörk, R., “Depth and All-in-Focus Imaging by a Multi-Line-Scan Light-Field Camera,” Journal of Electronic Imaging, Vol. 23, No. 5, Paper No. 053020, 2014.
[https://doi.org/10.1117/1.JEI.23.5.053020]
- Raytrix, “3D Light-Field Vision, Depth Sensors, Plenoptic Metrology,” http://raytrix.de, / (Accessed 10 NOVEMBER 2021)
-
Zeller, N., Quint, F., and Stilla, U., “Depth Estimation and Camera Calibration of a Focused Plenoptic Camera for Visual Odometry,” ISPRS Journal of Photogrammetry and Remote Sensing, Vol. 118, pp. 83-100, 2016.
[https://doi.org/10.1016/j.isprsjprs.2016.04.010]
-
Bodenstorfer, E., Fürtler, J., Brodersen, J., Mayer, K. J., Eckel, C., et al., “High-Speed Line-Scan Camera with Digital Time Delay Integration,” Proc. of the Real-Time Image Processing on International Society for Optics and Photonics, Paper No. 64960I, 2007.
[https://doi.org/10.1117/12.704516]
-
Jang, K.-W., Yang, S.-P., Baek, S.-H., Lee, M.-S., Park, H.-C., et al., “Electrothermal MEMS Parallel Plate Rotation for Single-Imager Stereoscopic Endoscopes,” Optics Express, Vol. 24, No. 9, pp. 9667-9672, 2016.
[https://doi.org/10.1364/OE.24.009667]
-
Yang, S.-P., Kim, J.-J., Jang, K.-W., Song, W.-K., and Jeong, K.-H., “Compact Stereo Endoscopic Camera Using Microprism Arrays,” Optics Letters, Vol. 41, No. 6, pp. 1285-1288, 2016.
[https://doi.org/10.1364/OL.41.001285]
-
Wei, S., Wang, S., Zhou, C., Liu, K., and Fan, X., “Binocular Vision Measurement Using Dammann Grating,” Applied Optics, Vol. 54, No. 11, pp. 3246-3251, 2015.
[https://doi.org/10.1364/AO.54.003246]
-
Niclass, C., Ito, K., Soga, M., Matsubara, H., Aoyagi, I., et al., “Design and Characterization of a 256 x 64-Pixel Single-Photon Imager in CMOS for a MEMS-Based Laser Scanning Time-of-Flight Sensor,” Optics Express, Vol. 20, No. 11, pp. 11863-11881, 2012.
[https://doi.org/10.1364/OE.20.011863]
-
Nandi, C., Tudu, B., and Koley, C., “Machine Vision Based Techniques for Automatic Mango Fruit Sorting and Grading based on Maturity Level and Size,” Sensing Technology: Current Status and Future Trends II, Vol. 8, pp. 27-46, 2014.
[https://doi.org/10.1007/978-3-319-02315-1_2]
-
Rivas-Lopez, M., Sergiyenko, O., Flores-Fuentes, W., and Rodríguez-Quiñonez, J. C., “Optoelectronics in Machine Vision-Based Theories and Applications,” IGI Global, 2018.
[https://doi.org/10.4018/978-1-5225-5751-7]
-
O’Mahony, N., Campbell, S., Carvalho, A., Harapanahalli, S., Hernandez, G. V., et al., “Deep Learning vs. Traditional Computer Vision,” Advances in Computer Vision, Vol. 943, pp. 128-144, 2019.
[https://doi.org/10.1007/978-3-030-17795-9_10]
-
Wang, J., Fu, P., and Gao, R. X., “Machine Vision Intelligence for Product Defect Inspection based on Deep Learning and Hough Transform,” Journal of Manufacturing Systems, Vol. 51, pp. 52-60, 2019.
[https://doi.org/10.1016/j.jmsy.2019.03.002]
-
Yang, J., Li, S., Wang, Z., Dong, H., Wang, J., et al., “Using Deep Learning to Detect Defects in Manufacturing: A Comprehensive Survey and Current Challenges,” Materials, Vol. 13, No. 24, Paper No. 5755, 2020.
[https://doi.org/10.3390/ma13245755]
-
Fu, G., Sun, P., Zhu, W., Yang, J., Cao, Y., et al., “A Deep-Learning-Based Approach for Fast and Robust Steel Surface Defects Classification,” Optics and Lasers in Engineering, Vol. 121, pp. 397-405, 2019.
[https://doi.org/10.1016/j.optlaseng.2019.05.005]
-
Mezgec, S. and Koroušić Seljak, B., “NutriNet: A Deep Learning Food and Drink Image Recognition System for Dietary Assessment,” Nutrients, Vol. 9, No. 7, Paper No. 657, 2017.
[https://doi.org/10.3390/nu9070657]
He received his Ph.D. degree in the department of Bio and Brain Engineering from Korea Advanced Institute of Science and Technology (KAIST), in 2021. Now, he is a senior researcher at Korea Photonics Technology Institute (KOPTI). His research interests include optical module design and evaluation for compact arrayed camera system.
E-mail: kisookim@kopti.re.kr
He received his Ph.D. degree in the department of Physics from Chung-Ang University in 2011. Now, he is working as a team leader at Korea Photonics Technology Institute (KOPTI). His research interest is optical coating and precision lens molding process for intelligent optical module.
E-mail: jpark@kopti.re.kr